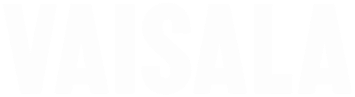
Better weather insights with deep learning
.png)
Silo AI uses inference acceleration with Intel® Advanced Matrix Extensions on 4th Gen Intel® Xeon® processors in flexible Deep Learning weather prediction solution for Vaisala Xweather
The accuracy of numerical weather prediction (NWP) models has increased steadily over the last decades thanks to better observational data as well as computational and scientific advances. However, there is still much room for improvement when it comes to predicting extreme weather events or local weather phenomena.
The main issues include:
- Improving forecast accuracy is not a simple problem. The weather is chaotic. Perfectly representing the laws of physics at different spatial and temporal scales using numerical models of the atmosphere and oceans is difficult to achieve.
- Initializing models with observational data from weather instruments is a complex process and restricts data fusion possibilities.
- Operating an NWP model requires long, costly simulation time on supercomputers, which puts real-time forecasts at risk.
Silo AI applies deep learning (DL) methodologies with accelerated inference leveraging Intel® Advanced Matrix Extensions (Intel® AMX). The solution fuses weather data from multiple sources for more accurate weather forecasts in Vaisala Xweather.
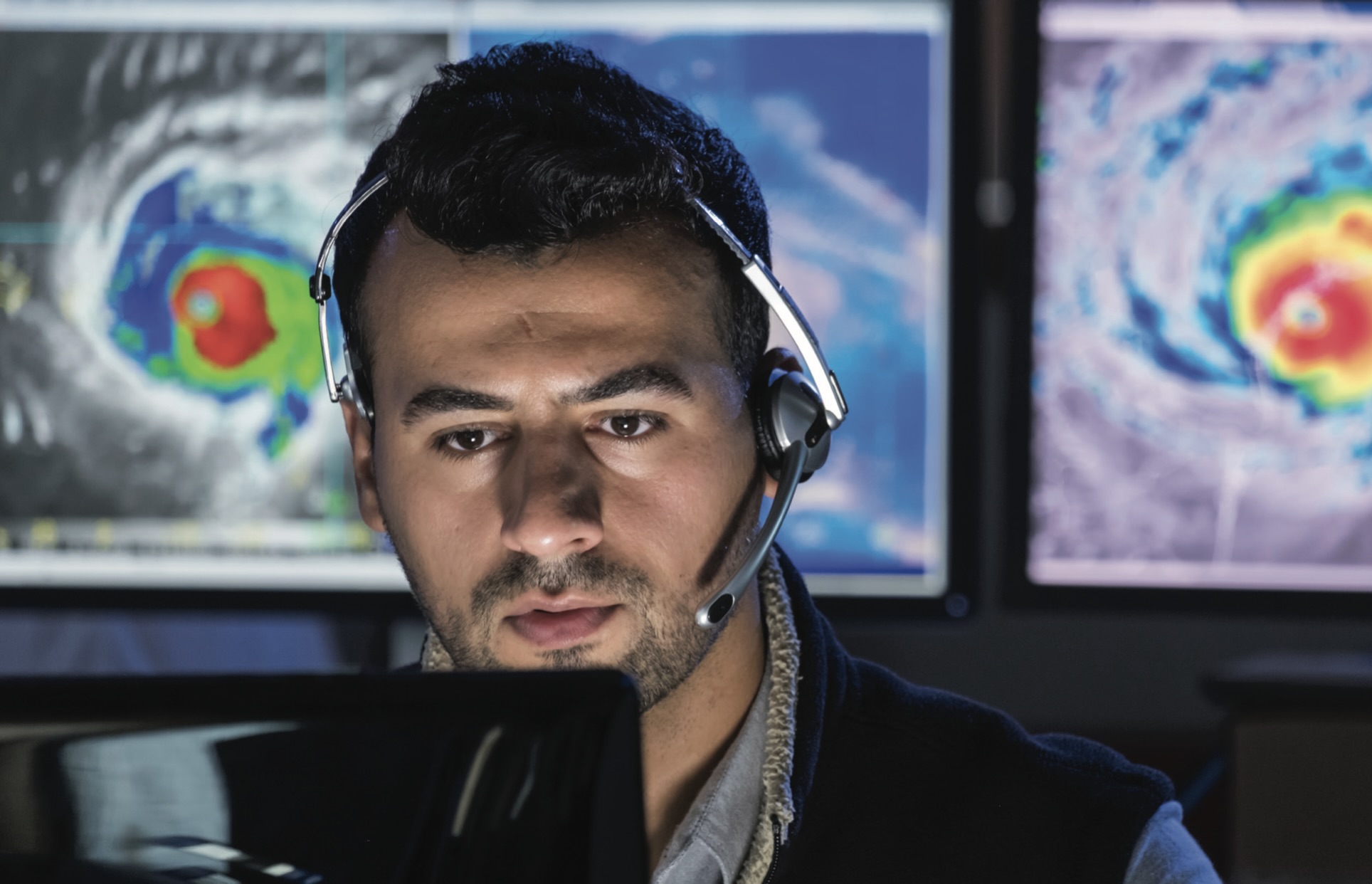
More Accurate Forecasts with Deep Learning-Based Data Fusion
Silo AI developed a flexible deep learning solution for Vaisala Xweather. The solution fuses data from weather sensors, remote sensing instruments, and other environmental data sources in an end-to-end learnable manner to provide novel insights. With this approach, the solution enhances the ability to detect and forecast atmospheric events. 4th Generation Intel® Xeon® processors with the new built-in deep learning accelerator, Intel AMX, optimizes inferencing performance.
Real-Time Insights at Scale
The solution leverages atmospheric and environmental data collected from multiple sources using cloud-based data processing pipelines. The data is continuously fed into a deep learning solution that fuses the data to produce weather insights. The AI-based weather analysis solution enables:
- Shorter lead times because the model output updates as soon as new input data is available. New insights can be issued much more frequently than with numerical weather prediction models.
- Versatile use cases from understanding local weather phenomena to continental atmospheric patterns.
- New possibilities to explore atmospheric events and improve predictive performance that are unattainable with current numerical weather prediction models.
Intel AMX on 4th Gen Intel Xeon Processors Improve Performance
The solution is deployable on Intel Xeon processors for cloud environments. Inference components use Intel® Distribution of OpenVINOTM toolkit for scalability. For optimal performance, deployment on 4th Gen Intel Xeon processors is recommended. These offer built-in acceleration with Intel AMX. The new accelerator engine significantly boosts inference capabilities, enabling faster and more actionable insights, while simultaneously lowering operating costs.
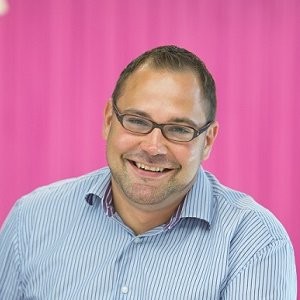
Samuli Hänninen
Head of Xweather
,
Vaisala
Optimizations Deliver Up To 5.5X Faster Processing
Silo AI completed benchmarking on Intel® DevCloud using multiple hardware architectures, leveraging acceleration and an OpenVINO-based model (see Figures 1 and 2).
The benefits of using the Intel Distribution of OpenVINO toolkit include:
- Up to 20 percent more FP32 throughput using OpenVINO on the 4th Gen Intel Xeon processors compared to PyTorch.
- Up to 550 percent more throughput using Intel AMX acceleration and OpenVINO on 4th Gen Intel Xeon processors compared to PyTorch.
- Up to 100 percent more FP32 throughput on 4th Gen Intel Xeon processors compared to previous generation processors.
- Up to 870 percent more BF16 throughput using Intel AMX acceleration on 4th Gen Intel Xeon processors compared to the previous generation processors.
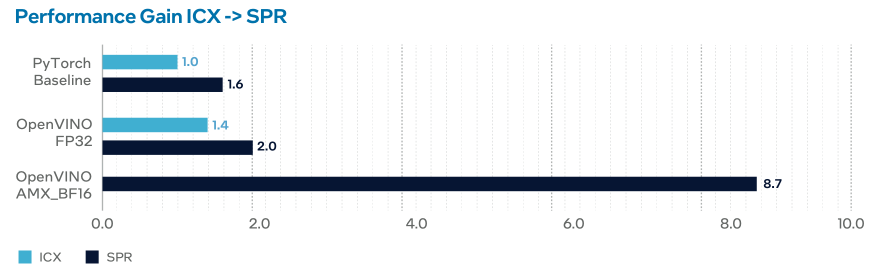
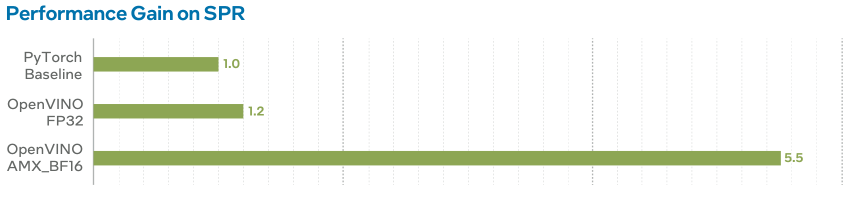
Conclusion
Silo AI has enhanced weather forecasting capabilities with deep learning and accelerated inference on 4th Gen Intel Xeon processors. By combining DL methods with multiple sources of observational weather data, the solution is able to fuse data and continuously learn to produce better insights into weather patterns. Using the Intel Distribution of OpenVINO toolkit and inference acceleration provided by Intel AMX, the solution achieves up to a 5.8X performance boost on the latest generation of Intel Xeon processors. The solution is built into Vaisala Xweather.
Ready to level up your AI capabilities?
Succeeding in AI requires a commitment to long-term product development. Let’s start today.
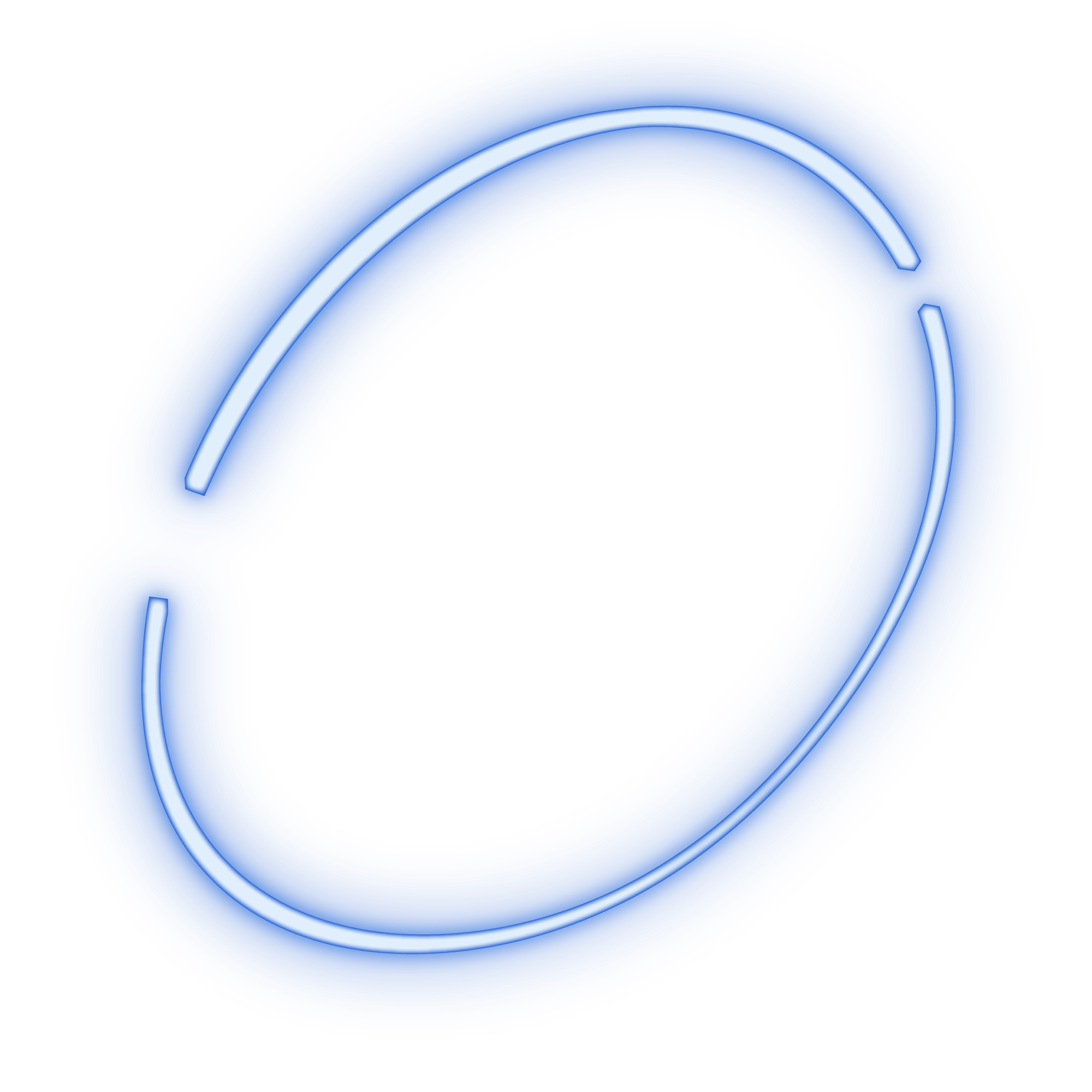