The Nordic State of AI
The annual Nordic State of AI report provides an overview of the use of artificial intelligence in the Nordic region, with the goal of offering business leaders, academics, policymakers, as well as anyone interested, a comprehensive view of the latest changes and developments in Nordic AI.
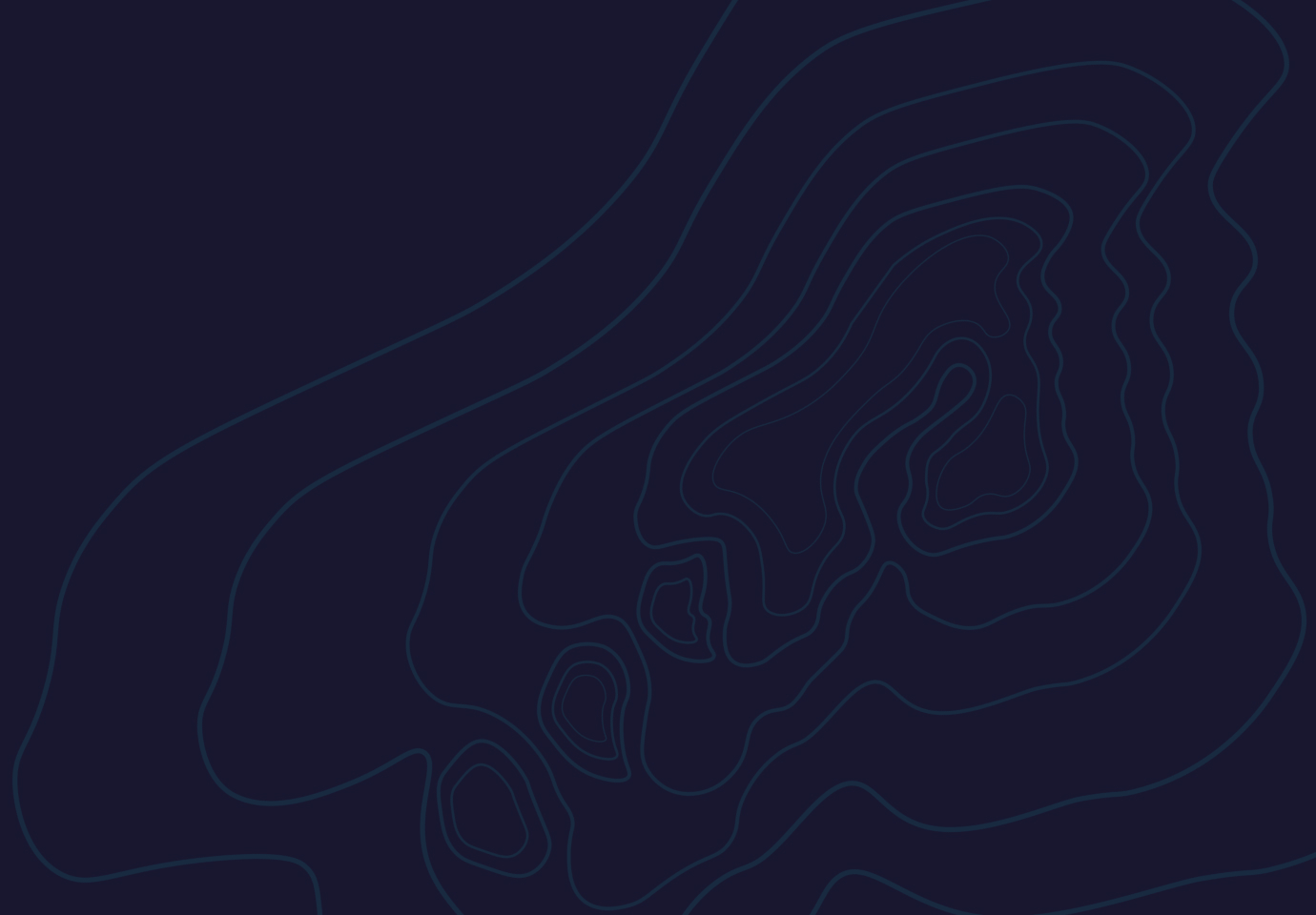
In collaboration with
Industrial incumbents embrace AI but
but face challenges in large-scale adoption

AI Adoption
Full-scale integration of AI into the core of companies continues to be an evolving challenge, increasingly an area requiring more investment.
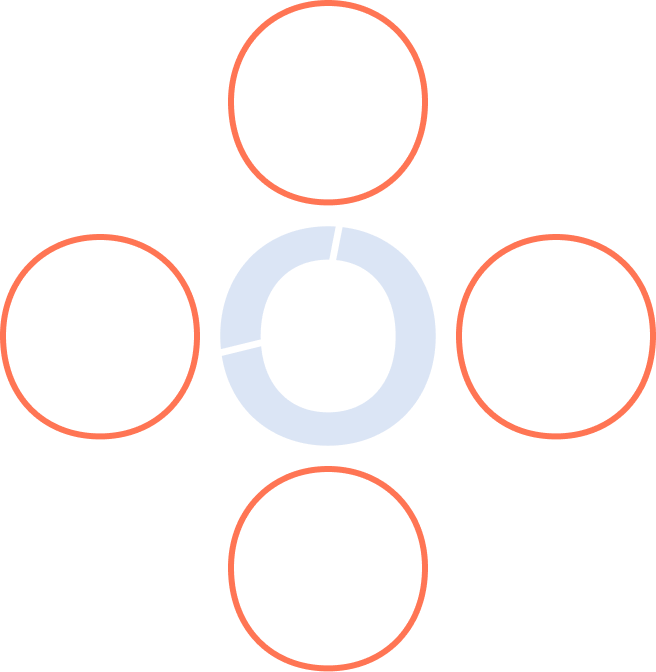
AI Talent
Talent is a growing bottleneck to AI adoption. Recruitment of AI talent is becoming increasingly critical as companies strive to scale their AI capabilities.

AI INFRASTUCTURE
For Europe and its forward-thinking companies, prioritizing investment in a flexible AI infrastructure is key, ensuring strategic readiness to harness AI's full potential.
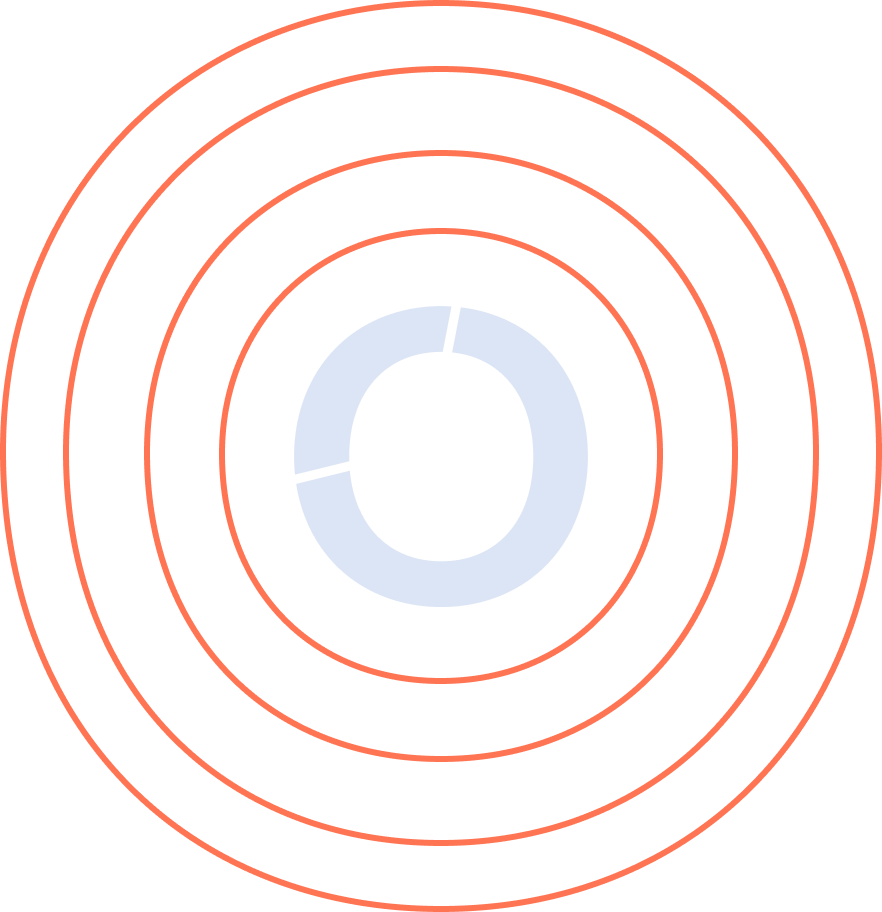
AI READINESS
The Nordic countries, and Europe at large, score high on AI readiness on a general level, but lag behind in the technology sector. This is why Europe needs software companies.

AI integration at the core still a challenge
Companies are primarily targeting shorter term efficiency improvements rather than deep integration of AI into the core of their business and products.
80% of respondents have started experimenting with ChatGPT-type LLM-products, and ¾ say that they use AI as part of their product or service, only 20% of respondents expect to invest more than €10 million in AI in 2024.
Talent is a growing bottleneck to AI adoption
The recruitment of AI talent is becoming increasingly critical as companies strive to scale their AI capabilities.
A majority of firms have identified a pressing shortage of skilled professionals, with 51.4% citing a lack of talent as a primary challenge, up from 35.3% in the previous year. This talent gap is most acute in high-demand roles such as data scientists, data engineers, and machine learning engineers.
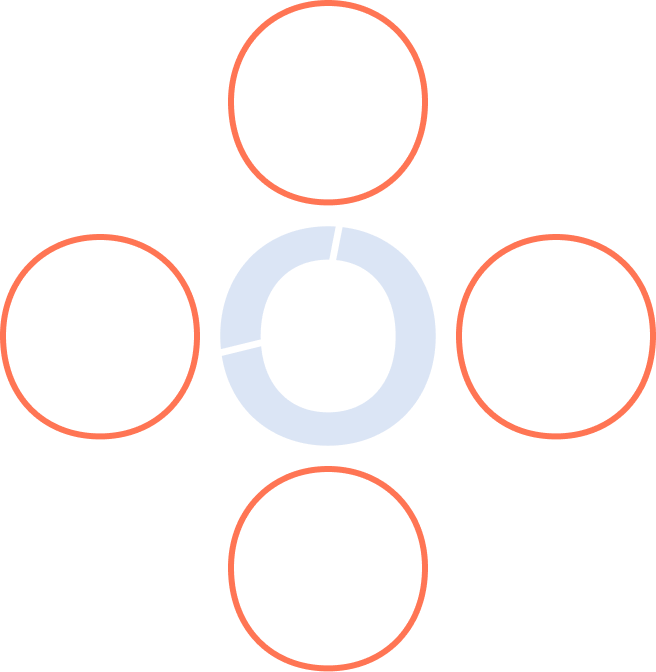

Prioritizing investment in AI infrastructure is key
Building a versatile and adaptive AI infrastructure in Europe is crucial.
AI infrastructure is key to developing AI-enhanced products and services tailored to European needs, embodying more than just computing power to include vital cultural, value-based and linguistic nuances.
Businesses will have to invest significantly not only in data quality and management, but also in the integration and running of AI models.
Europe lags behind in the technology sector
Europe should draw conclusions from earlier technological shifts like cloud computing and mobile technologies, where U.S. dominance led to missed strategic and economic opportunities.
To avoid a similar scenario in AI, Europe needs to actively shape its trajectory in line with its values and objectives, securing a competitive edge in the global AI arena and fostering technological progress that aligns with European strengths and market needs.
Oxford Insights Government AI Readiness Index lists all Nordic countries, except Iceland, in the top 15, with Finland as the only one in the top 10 with its 4th position. The index looks at three pillars: Government, Data & Infrastructure, and Technology Sector.
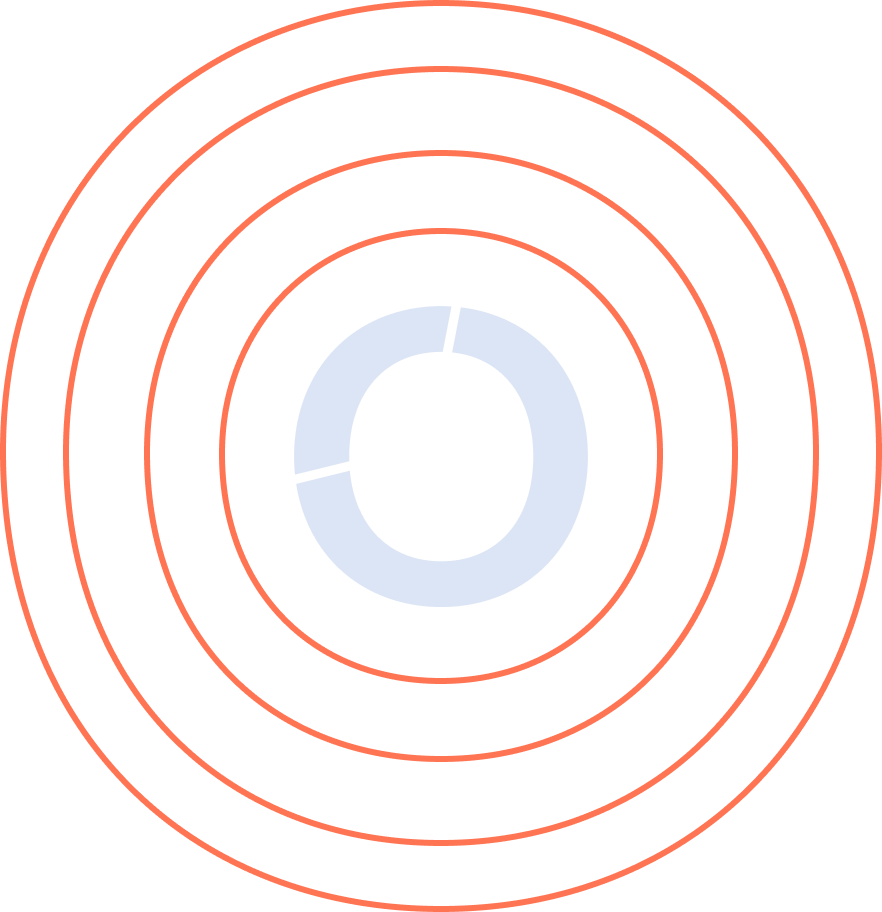
Read more unique interviews and all the key findings in the report.

AI Insights from the Nordics
Trends over time
When looking at which AI technologies companies use, the number of options have doubled from the first to the third report, reflecting the evolution, adoption and maturity of different AI technologies.
The most common answer for where AI is used has remained the same over the years; as part of a product or service. This year using AI as part of production of manufacturing processes has started to catch up.
For the first time, investing in training and competence development surpasses investing in recruiting new AI talent.
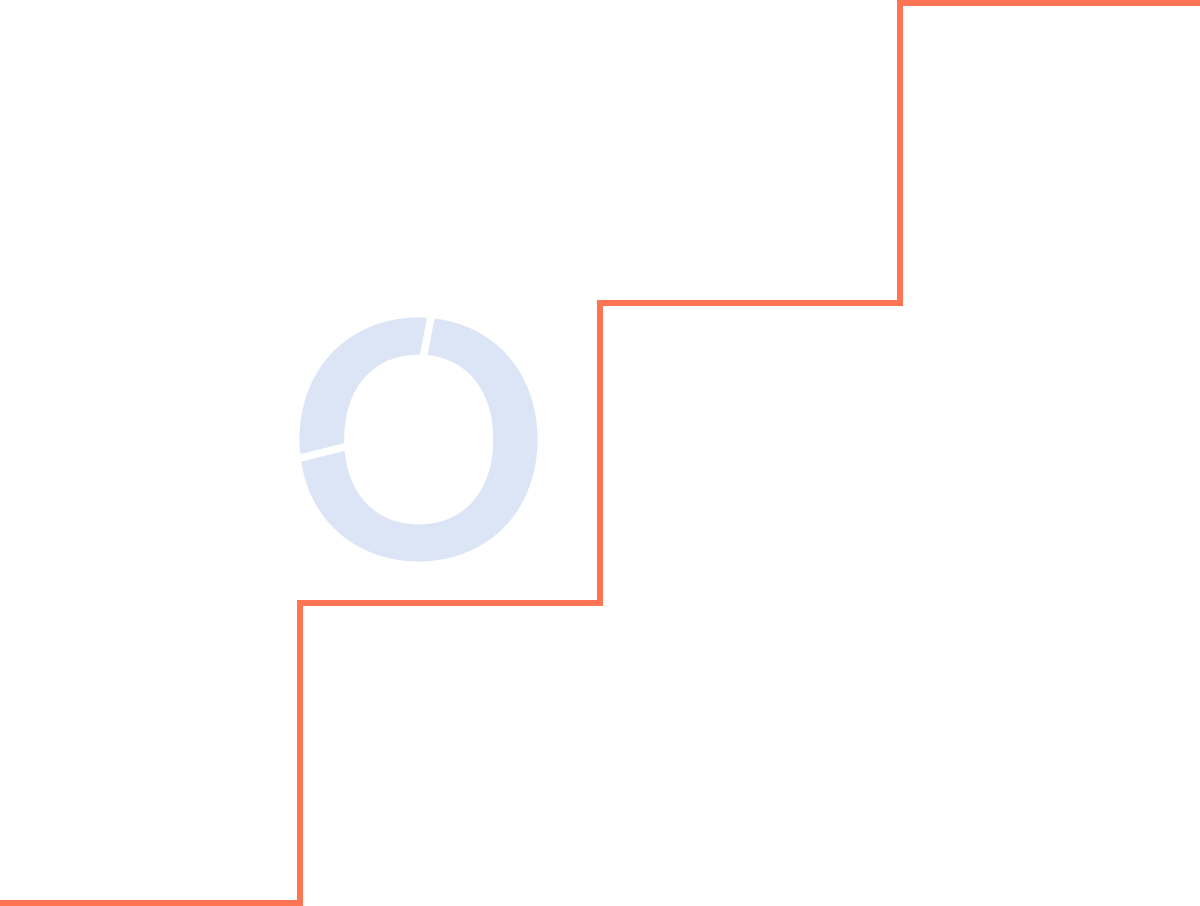
Get the Nordic State of AI report
Get the report now for a deep dive into the Nordic AI scene. Learn what is happening behind the curtains and immerse yourself in the latest trends and developments.