The automotive industry is increasingly implementing predictive maintenance and other diagnostics as add-on functionality to vehicles. In addition, the recent trend within the industry has been to create new business models and services supported by modern technologies, such as machine learning.
Our Lead AI Scientist at Silo AI Sweden, Professor Slawomir Nowaczyk, has been working with the Volvo Group in Gothenburg, building machine learning-based self-monitoring systems for trucks and buses. Today’s trucks are filled with IoT sensors that collect data, and computers that process it. Understanding the complex network of different components and sensors interacting with each other, is key in building a maintenance system around it. In this interview, Professor Nowaczyk shares some of the key learnings based on his work.
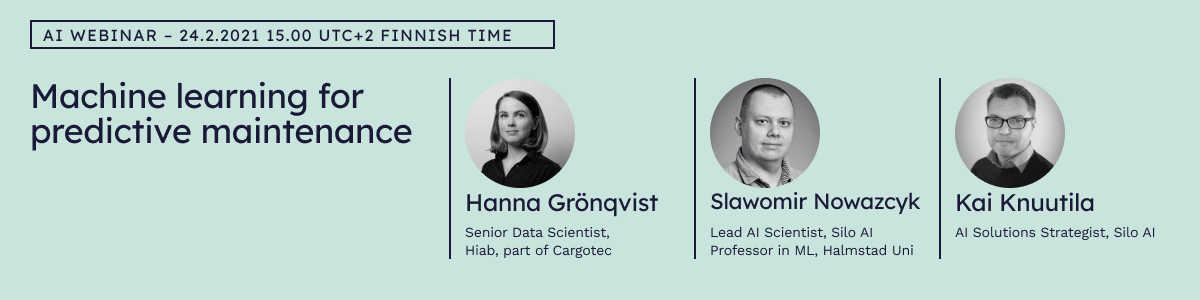
You've developed self-monitoring capabilities in vehicles in order to improve their ability of predict failures. What would be your advice for a company that wants to improve their predictive maintenance?
It’s important to start, so that you can learn by doing. The key is to begin small, and plan for continuous improvement in the longer term. Once the self-monitoring capabilities start bringing business value, it becomes a lot easier to put efforts into collecting more and higher quality data.
What would be a key learning from your various predictive maintenance projects?
The most important aspect is to set the expectations right: what is actually possible given the complexity of the equipment (and scenarios considered) in relation to the data available. It is tempting to set ambitious goals, but we should remember that in most companies we are virtually starting from scratch. Therefore, any solution, even an initially slightly inaccurate one, will be an important improvement, and we shouldn’t be afraid of starting this learning journey.
What do today's company’s often miss when trying to develop predictions of maintenance needs of their vehicles?
I have often seen confusion about actual goals. One example could be about historical repairs – should they be used as training for AI algorithms? If so, then the vehicle’s self-monitoring will try to replicate current human decision making – it will be very difficult for AI to improve upon that, and fix the mistakes people are doing today. In many cases there is an incentive for technicians to over-maintain equipment. It's important, but also challenging, for AI to compensate for it.
The second important problem is ensuring longevity of the solution. It is easy to train an AI solution on historical data, deploy it to make failure predictions, and replace components early enough to avoid failures. However, this might make further improvements to the system impossible, due to lack of new training data. With no run-to-failure examples, the AI can’t be adapted to new designs, or to changes in usage patterns, etc. As you can see, it is important to have a long-term strategy.
Data availability and machine learning are increasingly opening up possibilities to create smart predictions. What do you think is key in building a competitive edge through predictive maintenance?
Overall, the requirements on uptime, reliability and availability of almost all types of equipment is continuously increasing. As profit margins shrink and the economy is moving towards a more just-in-time way of operating, more industries will see the benefit of predictive and prescriptive maintenance, self-monitoring, etc. Data availability and popularity of machine learning are going to accelerate these trends. This story focuses on the automotive industry and vehicles, but lessons learned here are universal.
What do you think is a winning recipe in implementing predictive maintenance?
The biggest winners are going to be solutions that can be easily deployed in a flexible manner – few actors will have the resources to spend multiple years on developing predictive solutions. The key is to create a “good enough” solution that is automatically, or at least semi-automatically, created and adapts to changing environment, external conditions, and equipment model.
Thank you, Professor Nowaczyk for the interview! If you want to learn more, join our machine learning for predictive maintenance webinar on Feb 24.

Would you like to know more how Silo AI can help you build a solid machine learning-driven system for predictive maintenance? Get in touch with our VP, Sales & Business Development, Pertti Hannelin at pertti.hannelin@silo.ai or via LinkedIn.
About
Join the 5000+ subscribers who read the Silo AI monthly newsletter to be among the first to hear about the latest insights, articles, podcast episodes, webinars, and more.