The story of Silo AI has many beginnings, but one of the most foundational of all is the work we carried out, first with the European Central Bank (ECB), and later with many other central banks and financial supervisory authorities. An imperative part of this relates to the project with the ECB in 2014, in which we built an early-warning model to identify bank distress, with Tuomas Peltonen (Deputy Head, ESRB) and Jan Hannes Lang (Principal, ECB). The Machine Learning model we built then is still in use at the ECB, monitoring financial stability and helping to inform policy decisions. An article describing details on the model was recently published in the ECB Working Paper Series. Let’s look at how the model was created and what made it succeed in comparison with the input of 7000 experts.
Why the early-warning model was created
The global financial crisis brought a large number of European banks to the brink of collapse. There was a clear need for developing an early-warning model for European banks for three reasons: first, to avoid financial crisis for its real-economic costs. Historical evidence shows economic output losses from systemic banking crises of around 20–25% of GDP on average. Second, the euro area banking sector is crucial for the stability of the entire European Monetary Union. Finally, the banking sector is important in providing funds to the private sector, particularly to the small and medium size enterprises, which impacts the economies of the member countries and the whole Eurozone, and by extension, the lives and welfare of every European citizen. Having a model to identify vulnerabilities at an early stage allows policymakers to formulate micro- and macroprudential policies to prevent and mitigate the real economic impact of bank distress.
Measuring financial risk at two different levels
The model we developed for the ECB is called the Bank Early Warning Model (BEWM). It can be used to identify not only vulnerabilities in individual, systemically important banks, but also vulnerabilities that build up simultaneously across a number of banks at the country or Eurozone level. Moreover, it provides means to decompose model output to its contributing factors for model interpretability, as well as allows aggregating model output to assess the build-up of banking-sector vulnerabilities at the country or regional level. Embedded in a larger modeling framework, the technical solution itself is a fairly simple LASSO (Least Absolute Shrinkage and Selection Operator) based classifier built on a rich and unique data source of bank, banking-sector and macro-financial indicators and historical distress events.
An obvious challenge is that, in general, the outbreaks of banking or financial crises are inherently difficult to predict. We believe crises are oftentimes triggered by various, even unpredictable, shocks, but the build-up of widespread imbalances are identifiable. Hence, we focus on detecting underlying vulnerabilities, and finding common patterns preceding financial crises, rather than predicting the precise timing and shocks or other triggers causing a crisis. In our paper we focus on predicting vulnerable states (e.g. 8 quarters prior to distress events themselves), in which one or multiple triggers could lead to a systemic bank distress event.
Another challenge we had relates to so-called “black box” models, as policy tools and decision making for central banks needs to be transparent and accountability well defined. The ECB can’t set policy using opaque models with little or no understanding of causal or even statistical inference. This particular model had not only to be fully interpretable, but also familiar to existing ways of interpreting statistical models. The policymaker has to be able to justify a course of action based on an understanding of data, model and model output.Finally, our challenge was to get the model to real-time use. Operationalizing the model meant setting up data collection practices and creating and combining models, in addition to producing the outputs required to monitor highly vulnerable banks across Europe.
Comparing ECB Comprehensive Assessment and our model
Our model was compared with the ECB Comprehensive Assessment (CA) and Asset Quality Review, the so called “stress test”, which involved more than 7000 supervisors and regulators across Europe to prepare for the ECB’s new banking supervisory role as of November 2014. The below image shows a direct comparison of the results of the CA to the bank distress model, prior to the CA being published. As you can see, the work put in by our team of 3 people isn't far off from the CA.
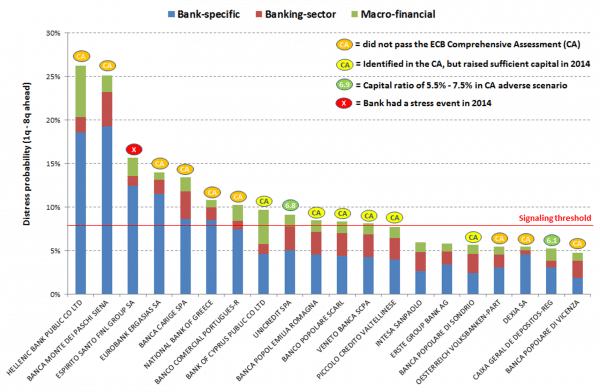
Powerful tools to help on big economic questions
As noted at the top of this piece, our bank distress model with both bank and country-level output is still in use, and is published regularly in the ECB Macroprudential Bulletin, as well as being used for various other policy purposes. Other previous coverage of our work with central banks includes VoXEU [1], Bloomberg [2], Forbes [3] and Central Banking [4]. The success with this model is not due to using the most advanced Machine Learning algorithms, but rather the way we balance model complexity, interpretability and other design factors to solve the problem at hand. We always design our tools and solutions to fit the required purpose, or to solve specific business or institutional needs.
Today, here at Silo AI we continue to provide solutions to central banks and financial institutions from the private and public sector, as well as across other industries. We build tools that free human experts from manually processing vast quantities of data, and models that support decision making in hugely complex environments. Machine Learning can be applied to large and even unstructured datasets - this is where computers can outperform humans. But the combination of computer processing power, careful model design and human expertise is the most powerful combination. That’s why we build systems that free people from analysis and processing work, empowering them to think creatively, and to act decisively with better information.
References
[1] The new ECB Macroprudential Bulletin, Vítor Constâncio. VoXEU, 25 April 2016. https://voxeu.org/article/new-ecb-macroprudential-bulletin
[2] The Financial Threats That Machines Can See, Mark Buchanan. Bloomberg, 18 April 2016. https://www.bloomberg.com/view/articles/2016-04-18/the-financial-threats-that-machines-can-see
[3] SWIFT Gets Academic, But Usefully Academic, Tom Groenfeldt. Forbes, 13 October 2014. https://www.forbes.com/sites/tomgroenfeldt/2014/10/13/swift-gets-academic-but-usefully-academic
[4] Teaching machines to do monetary policy, Daniel Hinge. Central Banking, 27 October 2017. https://www.centralbanking.com/technology/3270121/teaching-machines-to-do-monetary-policy
About
Join the 5000+ subscribers who read the Silo AI monthly newsletter to be among the first to hear about the latest insights, articles, podcast episodes, webinars, and more.