“With great power comes great responsibility”
AI-driven analytics for research or clinical practice
Among other fields already being affected, AI also strives to revolutionise the medical sector and healthcare. The amount of digitised health related data in the form of clinical records, genomic information, as well as diagnostic imaging tests of various modalities is rapidly increasing. This opens tremendous opportunities for AI-driven analytics to serve multiple purposes in healthcare, whether in research or clinical practice. Besides being one of the leading causes of death worldwide, cancer also creates significant financial losses affecting families, employers and the society overall. Due to a rapidly and globally ageing population, the economic burden of cancer is expected to increase significantly in the nearest future. In this regard, unleashing AI potential in medicine, and in oncology specifically, can dramatically improve treatment outcomes via providing more efficient, personalised and cost-effective treatments.
How AI could help to get a faster diagnosis
It is well known that diagnosing cancer at its earlier stages drastically improves chances for a cure. This suggests that accurate and early diagnostics are equally or even more important than developing novel treatment strategies. Most often the diagnosis of cancer is supplemented with visual examination of diseased tissue under the microscope by a pathologist. This procedure has been historically one of the major tools utilised in the diagnosis of solid tumours such as breast, prostate and colon cancers and remains still very important.Visual tissue assessment by a human expert, however, is semi-quantitative and subjective in its nature. As a result, a lot of inter- and intra-observer variations reduce reproducibility of the diagnostic conclusions, thus affecting clinical decision-making. AI-based approaches are already gaining momentum in tackling the issues of reproducibility and throughput in pathological tissue examination. This is achieved through ensuring improved accuracy in solving traditional tasks, such as cell counting, segmentation of certain tissue entities and classification of histological types. [gallery columns="2" link="file" size="medium" ids="https://silo.ai/wp-content/uploads/2018/10/cancer-diagnostics-AI-small-1-1.bmp|,https://silo.ai/wp-content/uploads/2018/10/cancer-diagnostics-AI-small-2-1.bmp|"]
IMAGE. Augmented Digital Pathology - Identification of necrotic cancer tissue areas on gigapixel images by Turkki et al.
More importantly, AI is capable to go far beyond automated assistance in histologic analysis. The vast information present within a tumour tissue specimen can be directly used to determine disease outcome, response to treatment, and even the need for surgical intervention or adjuvant therapy. For example, it has been shown that deep neural networks can predict disease outcome, i.e. patient survival directly from microscopic image of cancer tissue specimens only.
AI and decision-making in the medical field
When it comes to clinical decision-making that directly affects human lives, one must provide sufficient evidence to prove that the decision is reliable. With AI-based systems, providing such explanations is not always as straightforward as it is with more traditional machine learning models like linear regression or decision tree. However, deep neural networks are not black boxes as some people tend to perceive. When performing image analysis, various techniques allow to provide visual explanations of decisions from a deep neural network. An example below shows how a neural network trained to predict patients survival, learned which areas constitute cancer and focuses its attention on those regions when making predictions. The network was not trained specifically to localise cancer, yet it was capable to infer this important intermediate information while learning to solve more generic task.
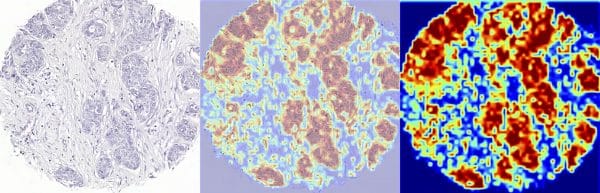
Image. Explanation of AI decisions - AI learns to identify and focus its attention on tissue areas that are most important for diagnosis and prognostication.
Finally, leveraging AI to integrate heterogeneous medical information all the way from raw texts of medical histories to genomic and imaging data will ultimately benefit patient care, scientific discovery and society overall. Find out more on the topic:
- Digital Pathology: Sometimes AI can outperform experts
- Towards the Augmented Pathologist: Challenges of Explainable-AI in Digital Pathology
Dmitrii Bychkov is going to host a Silo.AI Academy webinar in November about his research with AI and Cancer diagnostics. More information to follow! Make sure you don't miss it by following us or by signing up for our research community, Silo.AI Academy Slack.
About
Join the 5000+ subscribers who read the Silo AI monthly newsletter to be among the first to hear about the latest insights, articles, podcast episodes, webinars, and more.